Hi, I'm
Aaron Krauss
I'm a
I love being involved in my tech community
Here are some of the groups that I help manage and volunteer with:
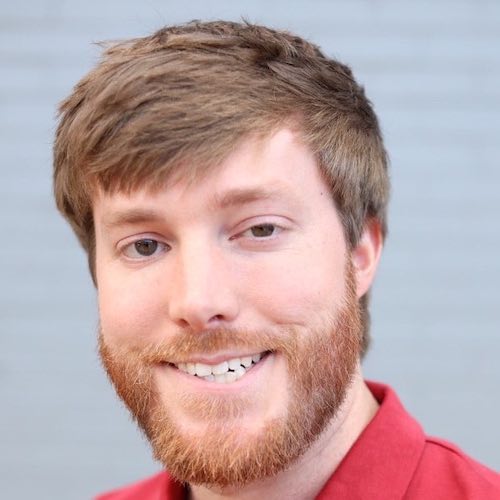
GET TO KNOW ME
Hi, I’m from Oklahoma City, OK, and I’m a technical lead at Clevyr with well over a decade of experience as a developer. I’m incredibly involved in my tech community, including organizing OKC WebDevs and helping to manage OKC Coffee and Code.
My favorite projects are those that are workflow-heavy involving Laravel, Vue, Tailwind CSS, SQL, Flutter, Ruby, Node, Go, Swift, Docker, CI/CD, and more.
Other than coding, I like brewing a hot cup of tea, making cocktails, longboarding, and hanging out with my wife Layla and sons Cyrus & Lucas.